Tools like ChatGPT might seem to speak your language, but they actually speak a language of probability and educated guesswork. You can make yourself better understood — and get more professional results — with a few simple prompting techniques. Here are the key ones to add to your toolkit.
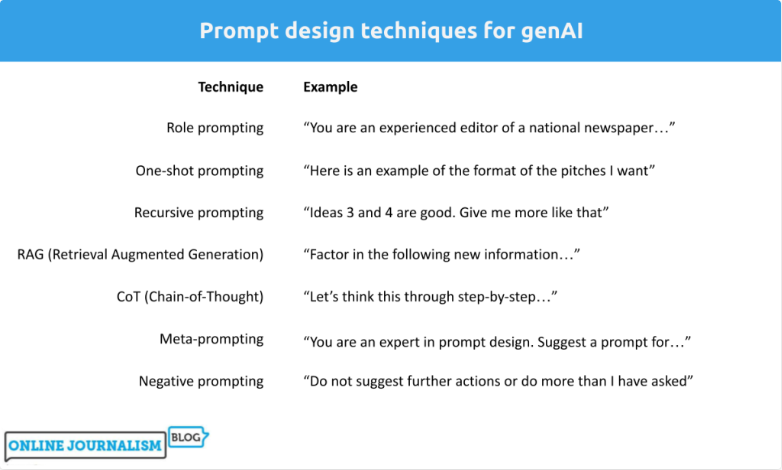
Role prompting
Role prompting involves giving your AI a specific role to play. For example you might say “You are an experienced education correspondent” or “You are the editor of a UK national newspaper” before outlining what you are asking them to do. The more detail the better.
There’s mixed research on the efficacy of role prompting, but at the most basic level providing a role is a good way of making sure you provide context, which makes a big difference to how relevant responses are.
That’s not just the context of a professional process (e.g. news editing, health journalism, etc.) but also the audience and the attitude you might be asking genAI to adopt.
For example, as genAI tools are often overly eager to please, talk too much, and lack a critical approach (more on this below), you can give them a personality which reduces this: “You are a health correspondent who is always carefully skeptical about any information that they receive,” for example, can help guide the AI to more critical suggestions for story ideas.
Recursive prompting
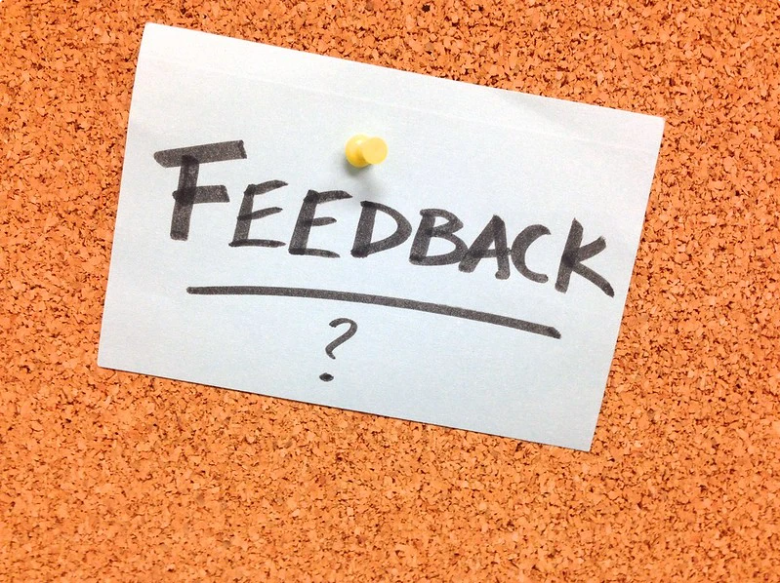
Recursive prompting simply means you don’t settle for the first response you get from your generative AI tool. Instead, you add feedback in follow-up prompts (“iteration”).
If you have asked for a number of ideas, for example, you might indicate which of those most closely meets your requirements, and ask it to generate more like that.
You can also provide more information on your request if it seems that the AI doesn’t quite understand what you mean.
Or if the AI’s responses tend to be longer than you’d like, you can iterate by telling it to be more succinct in its responses.
One consideration with recursive prompting, however, is the environmental impact. Using trial and error to arrive at a suitable response is likely to be more energy intensive than eliminating potential problems with a more carefully designed prompt to begin with.
One-shot, few-shot and zero-shot prompting
If you ask a generative AI tool to do something without showing an example of what you want, this is called "zero-shot prompting." It means you are relying entirely on the AI’s own knowledge of what might be meant by a "story" or a "pitch."
Providing examples of the kind of thing you want it to do is called one-shot or few-shot prompting (depending on whether you provide one example, or a few).
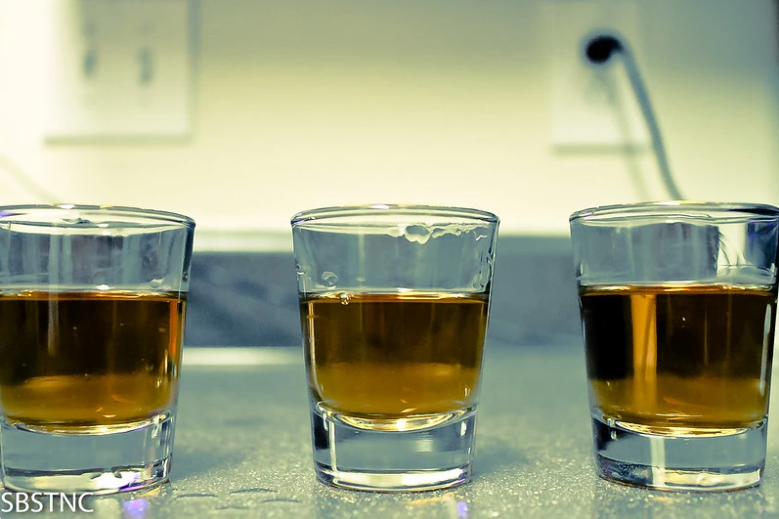
For something like story pitches where you want it to follow a particular template, one example (one-shot prompting) might be enough.
Few-shot prompting is often used for classification tasks, or where you might want to provide more freedom (such as multiple examples of what you mean by a good social media update).
Retrieval Augmented Generation (RAG)
AI’s strength — the fact that large language models (LLMs) have been trained on millions of lines of text — can also be its weakness. The "knowledge" in an LLM is wide but also limited: for example, it’s unlikely to know as much about recent events as it does about older events.
Retrieval Augmented Generation — augmenting the prompt with specific information — is a strategy for tackling this. The genAI platform Perplexity, for example, uses it to search for relevant and/or recent information to improve its response.
But we can "augment" our own prompts by choosing to include external information.
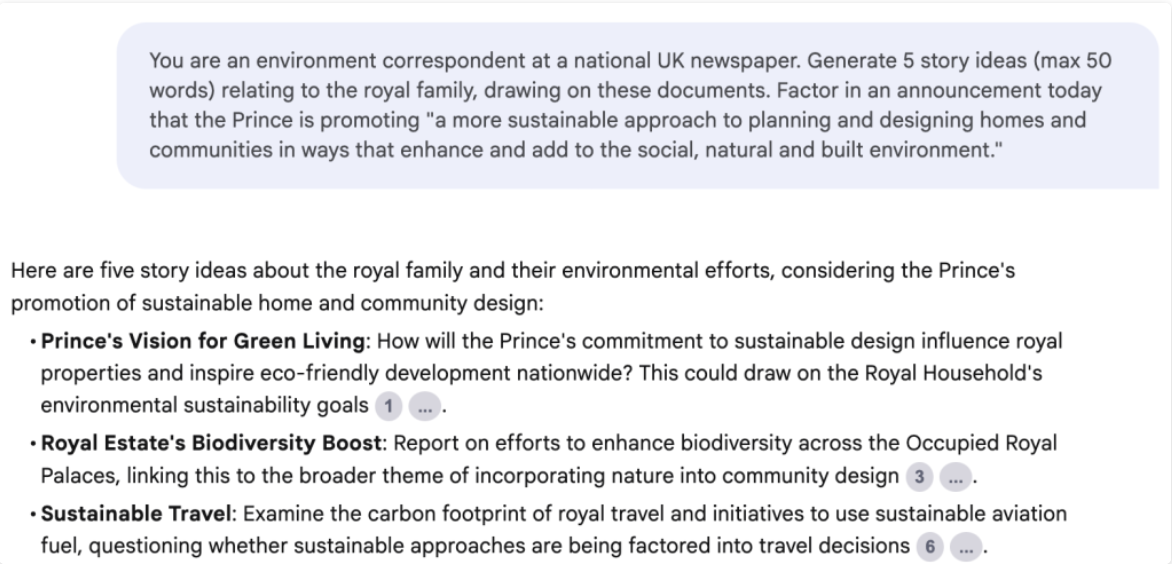
A common example is document analysis: instead of asking a large language model to generate ideas for stories about a company, we can upload their annual reports to "augment" its knowledge and direct its attention. Tools such as Google’s NotebookLM are designed specifically for such document-augmented prompts.
Another scenario where RAG can be useful is data journalism: by pasting a few rows of data, or accompanying notes on methodology to "augment" its understanding, you can help ground its knowledge in a more specific factual context. Specify that you don’t want it to perform any analysis, though, or it will try to over-deliver.
Uploading a publication’s style guide would be another example of RAG — but this experiment suggests it’s not very effective.
Chain-of-thought (CoT) prompting
Asking a generative AI tool to explain its thought process (its “chain of thought“) as well as the results can improve those results in certain scenarios.
In its simplest (zero-shot) form, CoT can be applied by adding “Let’s think this through step by step” to your prompt, but in journalism it’s likely you will need to specify a certain framework that you want it to work through.
For example, instead of asking it why a problem might exist, you can ask it to use the "5 Whys" approach, and explain its thinking. Or instead of asking it to come up with an idea for an investigation, you might explain the Story Based Inquiry method or the SCAMPER method, and ask it to break down its thinking along those lines.
You can also outline the steps you want to be taken: for example, for feature idea generation you might say: “Go through each of these steps in order: 1: identify who your audience is. 2: identify what their information needs are. 3: identify potential genres of feature you might write. 4: Come up with 3 ideas for each,” and so on.
Meta-prompting
If you are struggling with the "blank page" of an empty prompt, why not ask the AI itself to suggest prompts? This is called meta prompting.
A meta prompt might ask the AI to play the role of an expert in prompt design, and create a prompt to help solve a given problem or question.
Ideally you should describe what a good prompt looks like. You might provide examples.
And yes, at this stage we are getting very meta because we’ve just described a process which combines role prompting and one-shot prompting with meta prompting.
More advanced meta prompting can involve getting the AI to assess alternative prompts before identifying the best one. But meta prompting is perhaps most useful simply as a quick way of getting started. Then you can use recursive prompting to improve the results. But as with recursive prompting, meta prompting is likely to be more energy intensive than creating prompts independently.
Negative prompting
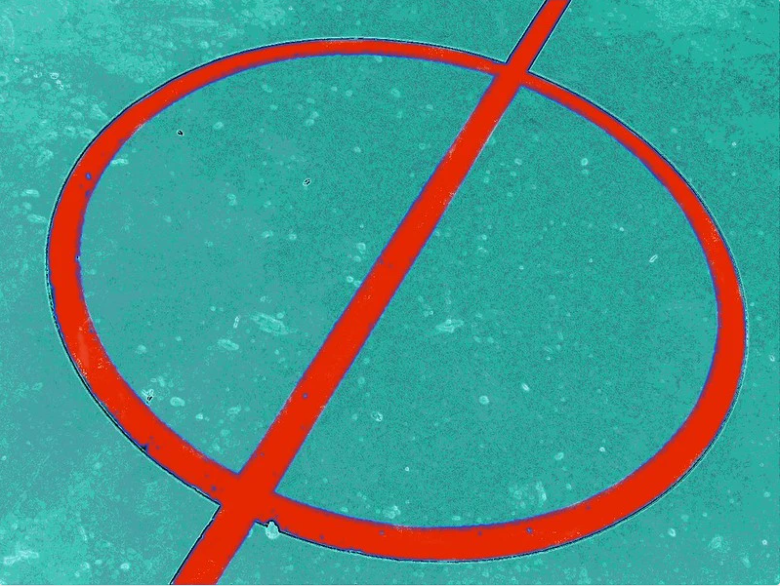
To get the best out of generative AI you need to curb its worst instincts. We know about its biases and its tendency to hallucinate, but then there’s also its tendency to be over-eager, put a positive gloss on things, and talk too much.
Negative prompting is all about what we don’t want the AI to do. Here are some examples:
- Throughout this conversation do not respond with anything beyond the scope of the request
- Do not try to please
- Do not suggest any further actions
- Avoid the tendency to be "gushing" and promotional. Remain skeptical and critical.
- Do not say too much – be succinct.
- Don’t try to answer something if you do not know the answer. Give an indication of certainty or uncertainty for your answer
- Don’t be biased. Take steps to address the fact that your knowledge reflects the bias of your training data
- Don’t make things up. Take steps to address the fact that you have a tendency to hallucinate material that is not true.
Adding these at the start of a conversation has a number of positive benefits. The most obvious is length and speed: responses are far less wordy and much quicker to read. It also reduces the inherent tendency for overreach. And if you want a really good response, try this follow-up prompt:
Update that response in the persona of a skeptical newspaper editor with years of experience dealing with misinformation, liars and crooks.
Photo by Google DeepMind via Pexels.
This article was originally published by the Online Journalism Blog and is republished on IJNet with permission.